72% of businesses worldwide have adopted artificial intelligence in at least one area of their operations, including user experience research. From automating mundane tasks to uncovering complex user insights, AI UX research has become a cornerstone for companies striving to design better, user-centric products.
At Eleken, a SaaS-focused UI/UX design agency, we see this transformation firsthand. In our daily workflows, AI has shifted from being a nice-to-have to an essential part of our research toolkit. Tools like Perplexity, ChatGPT, and Maze allow us to work smarter, automate repetitive processes, and extract actionable insights that would otherwise take hours of manual effort.
But here’s the thing: while the potential of AI in UX research is undeniable, knowing how to use it effectively is another story. Most articles either dwell on AI’s theoretical capabilities or present scattered tool lists without showing how they fit into the research process. That’s where our guide comes in.
We will walk you through every stage of the research process — Discovery, UX audit, Exploring, Testing, and Listening together with practical examples, expert insights, and specific AI applications at each step.
Disclaimer:
AI is not in a place to replace UX research — or research in any field, whether academic or industry — at this point. Instead, it serves as a tool for researchers. AI has significant limitations: it doesn’t reveal its methodology, making its results difficult to validate. Without transparency, its findings remain suspect. Moreover, AI cannot currently generate new ideas or insights beyond the data it is trained on.
Any company that believes AI can fully replace a skilled researcher is likely overlooking the importance of expertise and informed decision-making. As AI stands today, it’s a powerful assistant — but not a substitute for human researchers.
Now, let’s start with using AI for UX research at the discovery stage.
Discovery: building a strong research foundation
The discovery stage lays the groundwork for understanding your users — their behaviors, motivations, and pain points. This phase is crucial because it informs every subsequent step of the design process.
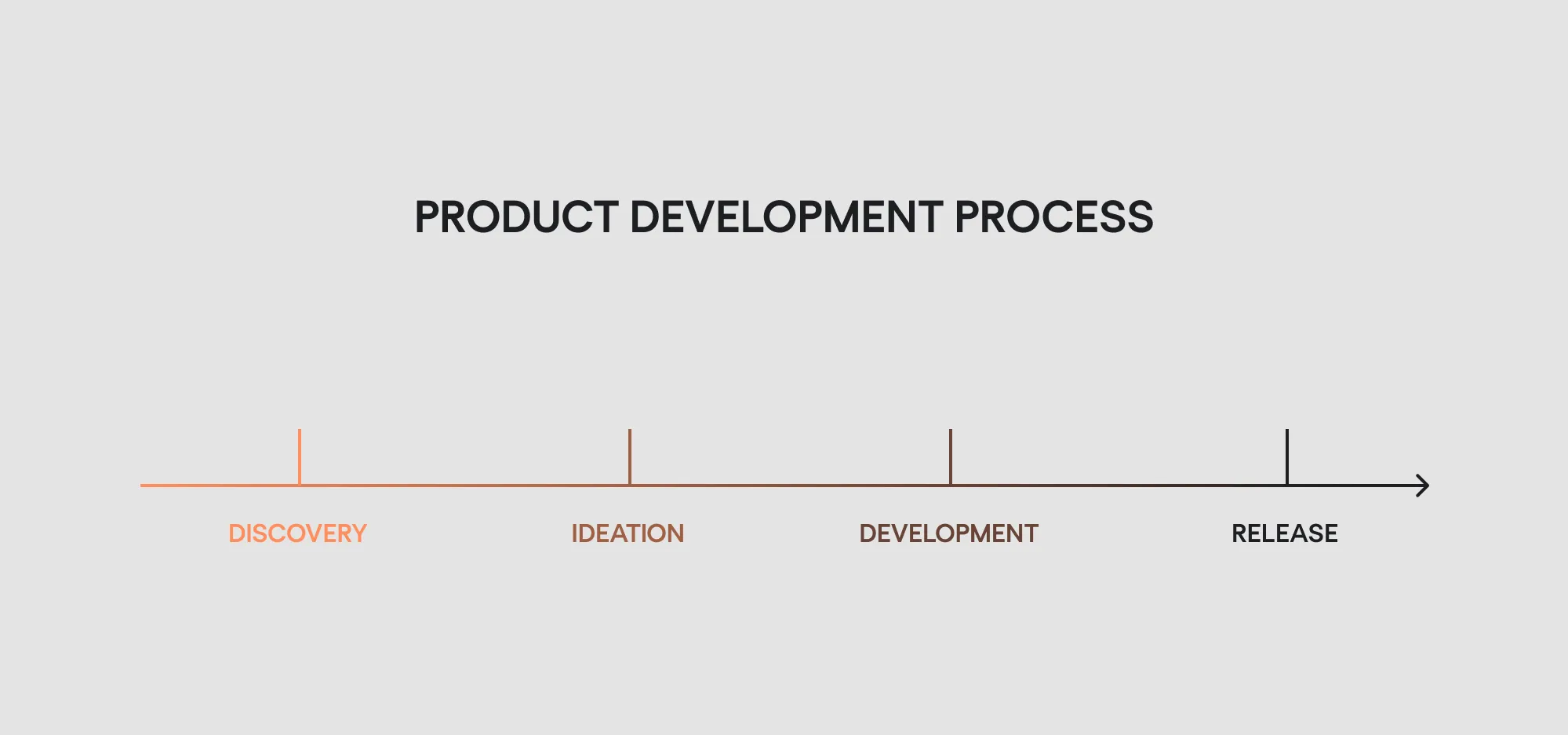
However, traditional discovery methods can be time-consuming and resource-intensive.
AI tools can streamline the process by automating data collection, transcription, and analysis, allowing researchers to focus on the bigger picture: uncovering actionable insights. Let’s explore how AI can enhance specific discovery tasks, from observing users in the field to gathering requirements from stakeholders.
Here are some common activities that may take place during the discovery stage:
1. Field study
Field studies involve observing users in their natural environments to understand how they interact with products and uncover behaviors and pain points. AI enhances this process by offering tools that allow researchers to process large amounts of qualitative data efficiently and focus on the critical insights they uncover.
Use case examples:
AI is able to process large amounts of data, summarize it, or find the needed piece of information in seconds. This way, AI in UX can be applicable to tasks like
- Real-time transcription for observations with tools like Otter.ai, or Loom AI transcription. These tools can save hours by transcribing things like session recordings and making summaries.
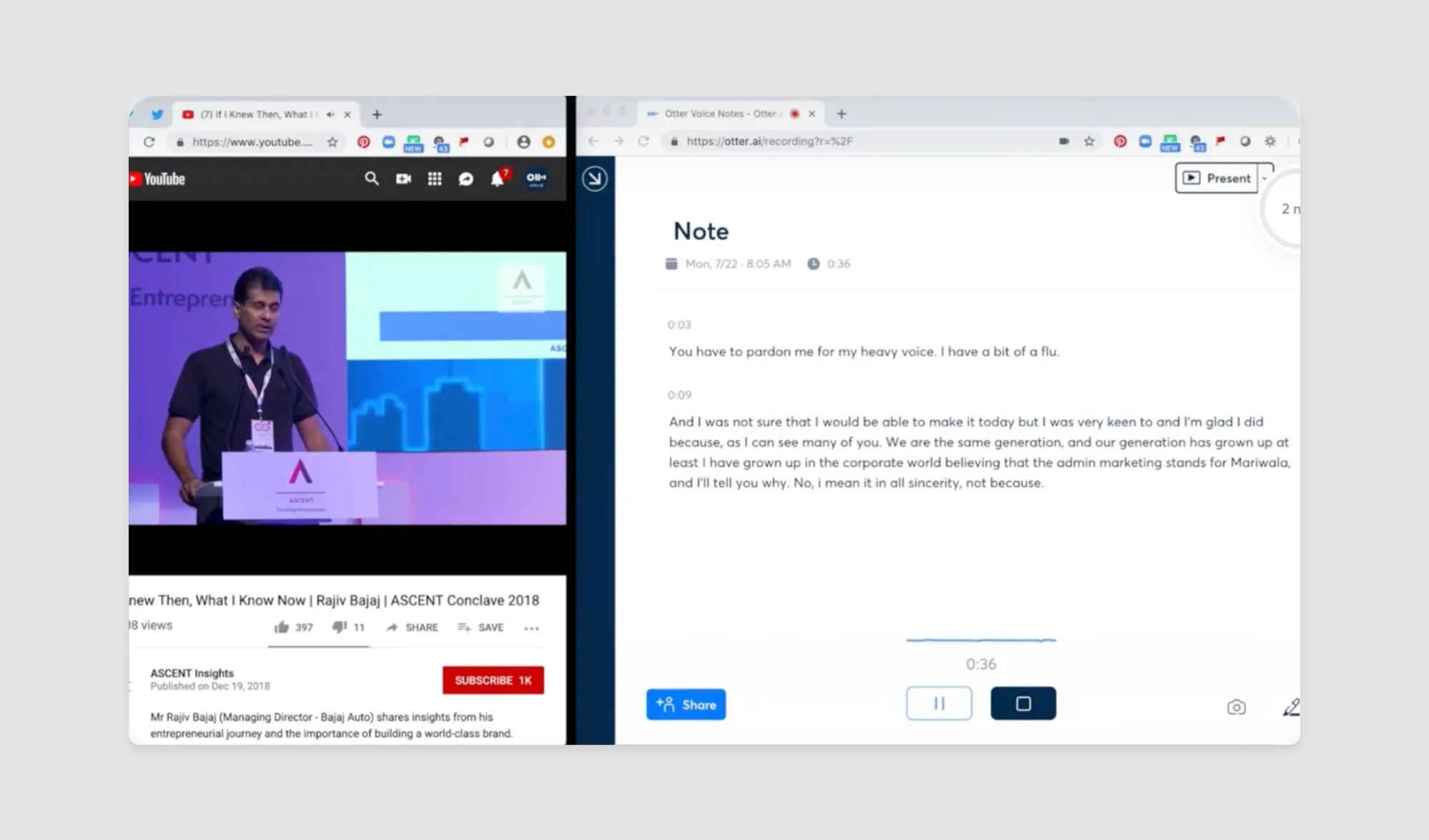
- Video/image analysis to extract meaningful patterns. An interesting case is using Affectiva AI tool to detect facial expressions, providing insights into user emotional responses. It can, for example, pick up subtle expressions of frustration that weren’t explicitly mentioned, allowing us to address specific pain points users encountered.
- Behavioral heatmaps to pinpoint areas of user interaction and identify trends, allowing for efficient analysis of complex datasets and understanding areas of confusion faster. For example, tools like Uizard.io or the AI Figma plugin Attention Insight can flag spots where users hesitate the most. Later, you will be able to check if it matches in user interviews.
- AI-driven tagging to organize and analyze large volumes of qualitative data, such as user feedback, interview transcripts, or usability testing notes. AI tools can cluster related user pain points, highlight frequently mentioned features, or detect emotional trends across user responses, For example, tools like FullStory and Hotjar are getting better at identifying friction points automatically, saving hours of manual review.
Practical tips:
- Pair AI transcription tools with manual validation to ensure critical context isn’t missed.
- Use behavioral heatmaps to narrow down problem areas before diving deeper into qualitative analysis.
- Combine AI tagging with human observation to balance automation and insight accuracy.
- Communicate clearly with participants about how data will be collected and analyzed to build trust and ensure compliance with privacy standards.
Limitations:
- AI lacks human intuition and struggles with understanding the broader context behind user behavior, leading to false positives or incorrect. For example, AI can tag behaviors as anomalies, but it still doesn’t understand the context behind why users do what they do. It often misinterprets deliberate actions as mistakes.
- AI research tools may misinterpret environmental noise or fail to recognize nuanced gestures during observations. For example, the mentioned tool Affectiva is good, but it sometimes misreads subtle facial cues, especially in diverse user groups where expressions can vary.
- Even with UX AI tools providing 100% accurate transcriptions, they can’t capture everything happening in a conversation. User interactions are more than just words — they include non-verbal cues, emotions, and context that transcription alone cannot convey.
- AI's suggestions are only as good as the data we feed it.
2. Diary study
Diary studies help researchers track user experiences over time, offering insight into long-term behaviors, frustrations, and emotions to see how the product fits into users’ lives. AI can simplify this process by analyzing large datasets and identifying recurring patterns.
Use case examples:
- Sentiment analysis with NLP tools helps detect emotional trends in diary entries.
They allow to spot recurring frustrations in user logs, helping to redesign workflows. For example, Google AutoML could be used to track participants' emotional states throughout the diary study. Researchers can identify how users' moods evolve over time in response to a product or service. This helps determine which features contribute positively or negatively to their experience, giving a detailed, sentiment-based view of the user journey. - Summarization and structuring to condense long-term diaries into actionable highlights. ChatGPT is a great tool for UX researchers when you learn how to use it. It can create weekly summaries of diary entries. For example, if participants submit entries daily, ChatGPT can summarize the content to highlight any emerging themes or significant changes in perception over time.
Here’s one more way to use ChatGPT from our UI/UX designer Nazar Neshcheret:
If I have a spreadsheet table with quantitative data, I upload it to chatGPT, and it calculates all the key values and even draws conclusions.
- Behavioral analysis. There are AI tools known for analyzing user behaviors in sessions serving like digital diary (if the diary study is conducted through a web or app interface). For example, researchers could use FullStory to determine which parts of a diary interface are confusing or difficult to use and to understand users’ behavior while they’re filling out diary entries. LifeData provides an "eDiary" mobile app for flexible diary study designs, including event-based reporting and daily diary studies.
Practical Tips:
- AI tools work best when participants provide rich content. Just as with interviews, where AI summarization is more effective with detailed responses, encouraging participants to provide more context and detail in diary entries will lead to more meaningful AI-generated insights.
- As community members highlighted, AI should ideally supplement, not replace, human analysis. Reviewing AI-generated summaries and sentiment analysis outputs is crucial to ensure nothing significant is missed. In diary studies, this is especially important because context across multiple entries could contain subtle cues that AI may miss.
- One effective way to use AI is to help prompt diary entries. This can help generate richer diary data that is easier for AI to analyze.
- Always ask AI to provide you with sources and double-check them.
Limitations:
- AI has limitations when it comes to capturing the nuanced connections across multiple diary entries over an extended period. Diary studies inherently require understanding how user experiences evolve, and AI often struggles to build a holistic picture over time without explicit links between entries.
3. User interviews
User interviews are critical for uncovering user motivations and pain points. AI user research can assist in creating targeted interview scripts, transcribing conversations in real time, and summarizing key insights post-interview. By automating these aspects, researchers can focus on building rapport with participants and diving deeper into qualitative data.
Use case examples:
AI can streamline user interviews in several ways:
- Transcription and summarization: This point doesn’t differ from the one we had on the Field study part. Tools like Otter.ai or Notably AI can transcribe conversations in real-time, allowing to skip re-listening to hours of audio and get right to analyzing insights.
As for summarization, our designers utilize AI a lot for this purpose:
For example, when we conduct an interview, there are AI Notes that will highlight key points. Even better, Loom screen recorder that we use a lot has this feature built into the product. – Nazar Neshcheret.
- Theme identification: ChatGPT or Claude AI can process multiple interview transcripts to identify recurring themes or pain points.
"I guess it could also summarize learnings across many interviews and recommend features or something, but I haven’t gotten that far yet," said one Reddit user. - Follow-up question generation: AI can craft customized UX research interview questions based on the research goals and user profiles.
"I’m using it to generate interview questions based on the mom test and info I feed it about each contact and the broader goal of the project," said a Reddit community member. - Moderated and unmoderated analysis: AI tools like Claude AI are used to analyze sessions in different contexts, both live and recorded. You can try using this tool both in moderated and unmoderated interview analysis.
As well, you may check this video by Dwarkesh Patel to get interesting insights on how to work with Claude:
Practical Tips:
- Use AI summaries as a starting point, but cross-check results to ensure critical nuances aren’t lost.
- Feed AI tools relevant project context or user information to tailor outputs. For instance, uploading project goals or interviewee details can make AI-generated insights more accurate.
- After your first round of interviews, use AI tools to identify gaps in data and generate targeted follow-up questions for subsequent sessions.
Limitations:
- Automated summaries may miss subtle, contextual points that are important for qualitative analysis.
"AI interviewers — for me it misses some of the point. I think the experience of learning from a participant and navigating conversations with them is often where seeds of insight lie, for an individual researcher and the whole product team," shared a Reddit user.
- AI doesn’t generate ideas: AI can synthesize information, but the deeper understanding of users’ activities and intent in context — where true insights come from — still depends on human interpretation.
4. Stakeholder interviews
Stakeholder interviews ensure research goals align with business objectives and internal priorities. AI supports this process by helping prepare structured questions, transcribing discussions, and analyzing sentiment to highlight recurring concerns or priorities. This approach makes it easier for researchers to extract actionable insights from conversations with business leaders.
Use case examples:
AI can support stakeholder interviews in similar ways as we discussed with user interviews, so let’s briefly mention several points:
- Structured question preparation: AI tools like ChatGPT can create tailored interview questions based on the project’s goals and prior feedback.
- Sentiment analysis to highlight key themes can analyze interview transcripts to detect recurring concerns or emotional patterns. Check out Dovetail AI to automatically detect positive and negative sentiment in your transcripts and notes, track patterns and trends, and identify recurring pain points, providing rich insights into your users’ emotions and attitudes over time.
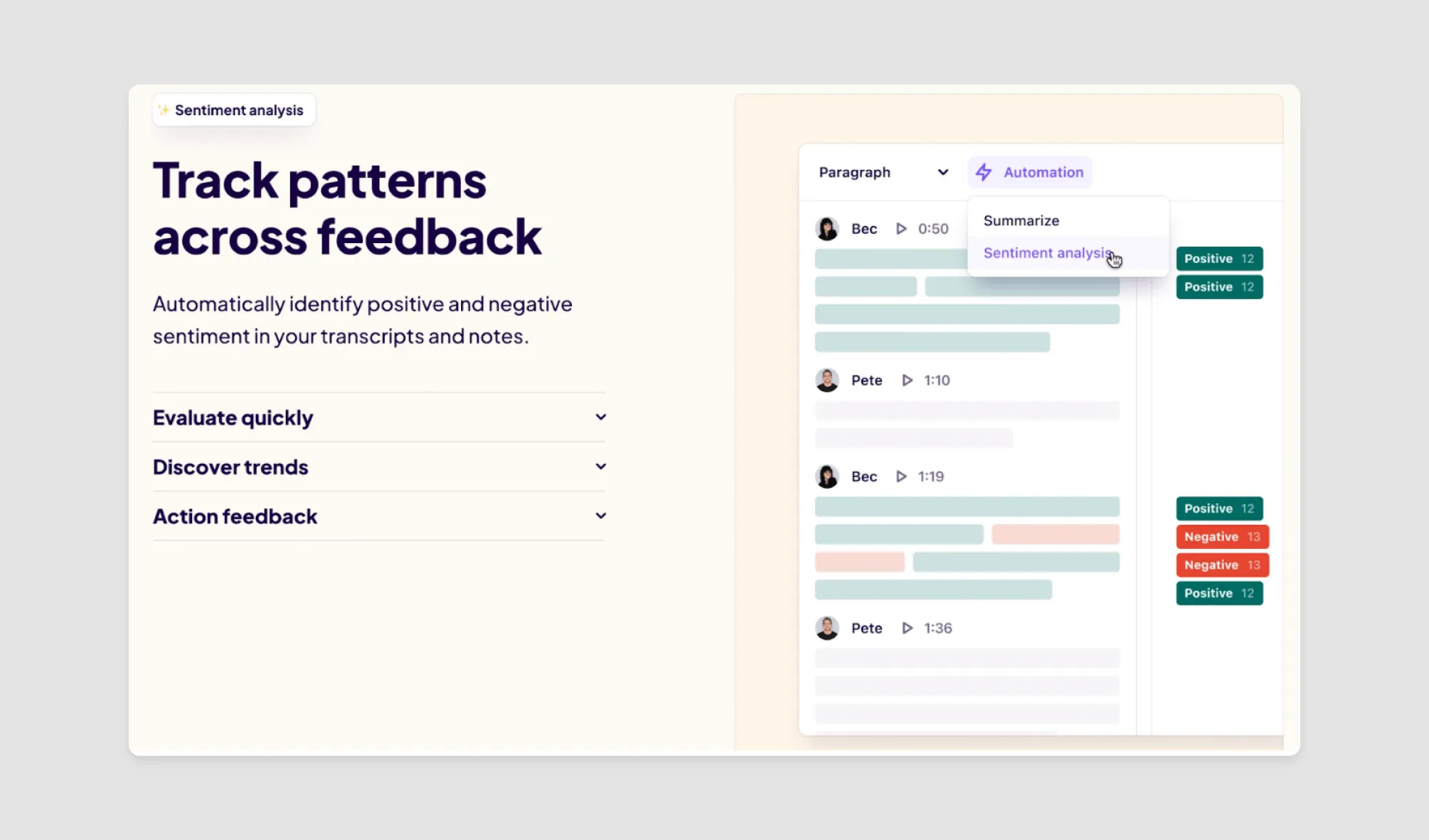
- Automated meeting summaries: AI tools like Notion AI or Read AI generate summaries of stakeholder meetings, highlighting action items and discussion points.
Practical tips:
- Use AI tools to identify common themes across multiple stakeholder interviews. Focus on aligning these goals in the research to ensure cross-functional support.
- While AI can process transcripts and detect themes, it’s essential to engage stakeholders in live discussions to explore motivations behind their priorities.
- Presenting AI-generated insights in the form of charts, diagrams, or summaries can help communicate findings more effectively to stakeholders.
Limitations:
- AI-generated summaries may overlook critical context or prioritize less relevant information, requiring careful review.
- Some stakeholders may be wary of AI tools, either due to privacy concerns or skepticism about their accuracy. Always ensure transparency about AI’s role and limitations.
5. Requirements gathering
Requirements gathering consolidates user and stakeholder needs into actionable deliverables. AI assists by summarizing lengthy documents, identifying gaps or contradictions, and organizing requirements based on prioritization logic. This ensures clarity and alignment across teams, enabling faster decision-making in the design process.
Use case examples:
AI can assist with requirements gathering in the following ways:
- Summarizing feedback: Tools like ChatGPT and Notion AI can extract key points from long meeting notes or documents.
- Creating compelling reports: AI can help UX researchers craft better reports and presentations by automating visual and textual summaries.
- Organizing requirements into hierarchies: AI-powered tools like Perplexity AI and ClickUp AI can cluster related requirements into categories or priorities. This helps teams visualize deliverables in a structured way, ensuring nothing critical gets lost. As well, AI-powered whiteboards such as Miro or Figjam AI can be very helpful here.
Below you can see an example of how our designer Daria Kornienko used Figjam AI to structure information about user persona into easy-to-comprehend text.
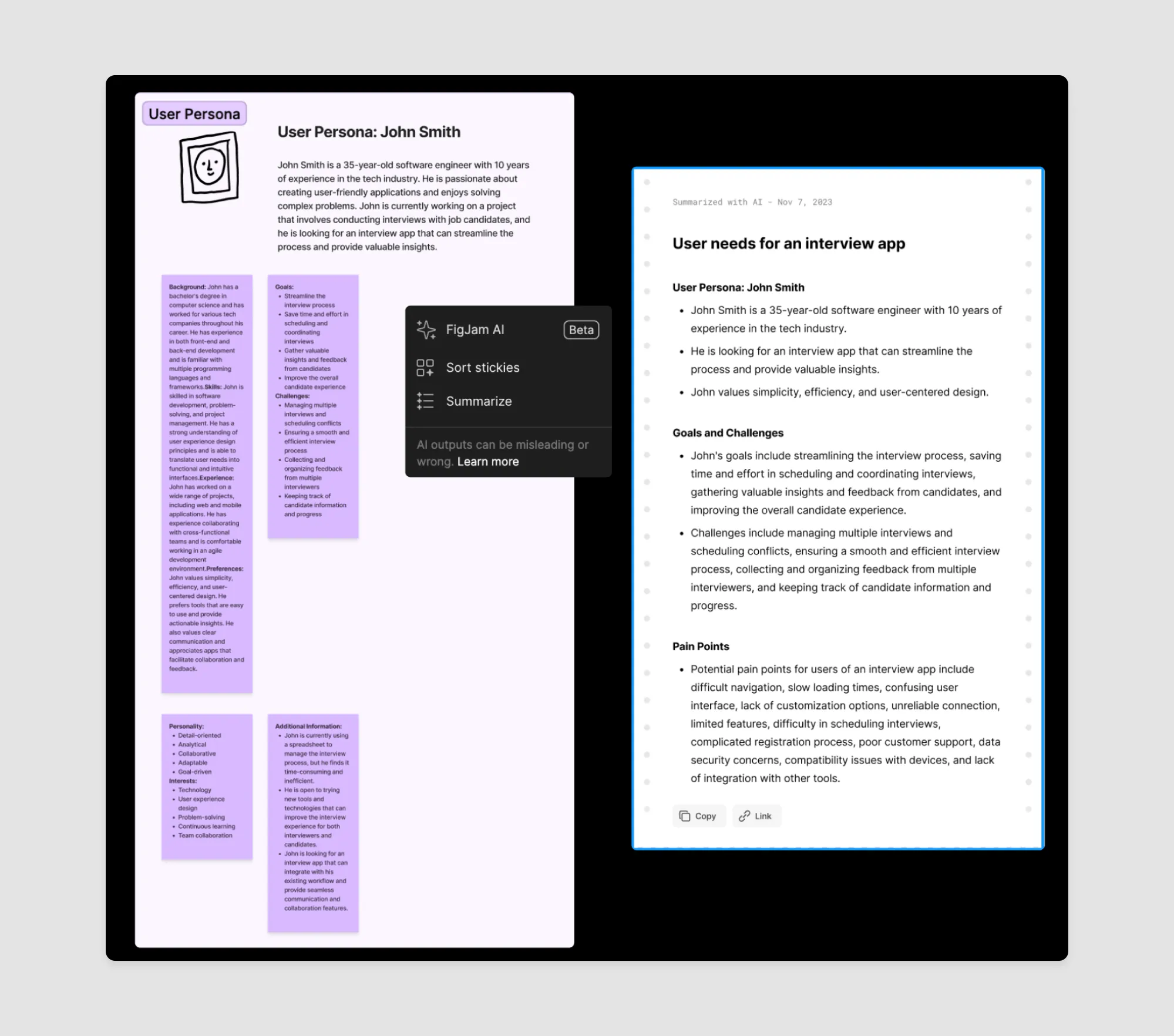
- Summarizing quantitative research data: If requirements include numerical data or survey results, AI can aggregate and analyze findings to provide key takeaways.
Practical tips:
- Use tools like Notion or ClickUp to keep user feedback, stakeholder inputs, and research notes in one place. AI tools perform better when they can access well-organized datasets.
- Use AI as a first pass for summarization or organization, but always cross-check with team members to ensure all key requirements are captured accurately.
- Tools like Miro AI or FigJam AI can create visual maps of requirements, which help teams understand priorities and dependencies more effectively.
Limitations:
- AI tools may oversimplify complex requirements or fail to account for implicit priorities.
"It’s like asking a junior researcher to synthesize some text for you without telling them what the point of your study was. You will really need to review the data yourself to determine whether you agree with an analysis and if themes were missed," said a Reddit community member. - If inputs are incomplete or inconsistent, AI results may be unreliable.
- AI can highlight contradictions in requirements but cannot resolve them — this still requires human judgment and collaboration.
- While AI can identify "themes" in data, it cannot fully understand the broader goals or nuances of your research.
"ChatGPT is just not great at thematic analysis yet. It can spit out themes, but it doesn’t have the full context of what you’re looking for with research, which can dramatically alter the themes it looks for," cautioned a Reddit user.
Now, let’s move to the next stage of UX research.
UX Audit: enhancing evaluations with AI
A UX audit evaluates the usability and effectiveness of a product, identifying areas that need improvement. It’s a diagnostic process that helps ensure your design aligns with user needs and expectations. Traditionally, UX audits rely on a combination of manual reviews, analytics, and heuristic evaluations, which can be time-intensive and prone to human error.
AI in UX audits can streamline and enhance the process by automating data analysis, identifying usability issues, and providing actionable insights.
1. Automated heuristic evaluation
Automated heuristic evaluations use AI to simulate user interactions and identify usability issues based on predefined rules or heuristics. AI tools analyze interfaces, detecting problems such as confusing navigation, inconsistent design patterns, or inaccessible elements.
Use case examples:
- Evaluate existing designs. Nazar Neshcheret, a designer from Eleken, shares:
During the audit, I often take screenshots and ask ChatGPT to analyze these pages according to heuristics. However, remember that AI does not have enough expertise for this. Screenshots are still not very well recognized, and ChatGPT misses a lot of points. However, this method holds promise for the future.
- Export site to Figma: With tools like Builder.io, html.to.design you can input a website URL, and they will export its structure directly into Figma. This makes it easy to analyze layouts, experiment with concepts, and reimagine designs efficiently (especially for old sites with no documentation).
Practical tips:
- Combine AI evaluations with manual heuristics: Use AI as a starting point to identify potential issues, but follow up with manual reviews to ensure findings are aligned with user context.
- Set clear parameters: Provide the AI with clear heuristics or benchmarks relevant to your product’s audience to improve accuracy.
- Validate AI insights with real users: Incorporate usability testing alongside AI findings to confirm whether flagged issues genuinely impact user experience.
Limitations:
- Contextual blindness: AI may flag issues that don’t reflect real-world user needs. For example, it might identify a low-contrast button as a problem, even if it’s rarely used in practice.
Here’s a comment from a Reddit community that proves this point:
AI often misinterprets what’s important to users. For example, it flagged a footer link that barely anyone clicked, wasting our time on an irrelevant fix.
2. Analytics review
Analytics reviews analyze user behavior data to uncover bottlenecks and friction points. AI-powered platforms like Google Analytics and Amplitude use machine learning to identify patterns, such as drop-offs in user flows or frequently abandoned tasks. These insights help prioritize improvements based on user behavior.
Use case examples:
- Traffic and behavior analysis: Tools like Heap can highlight user drop-offs through its funnel analysis feature.
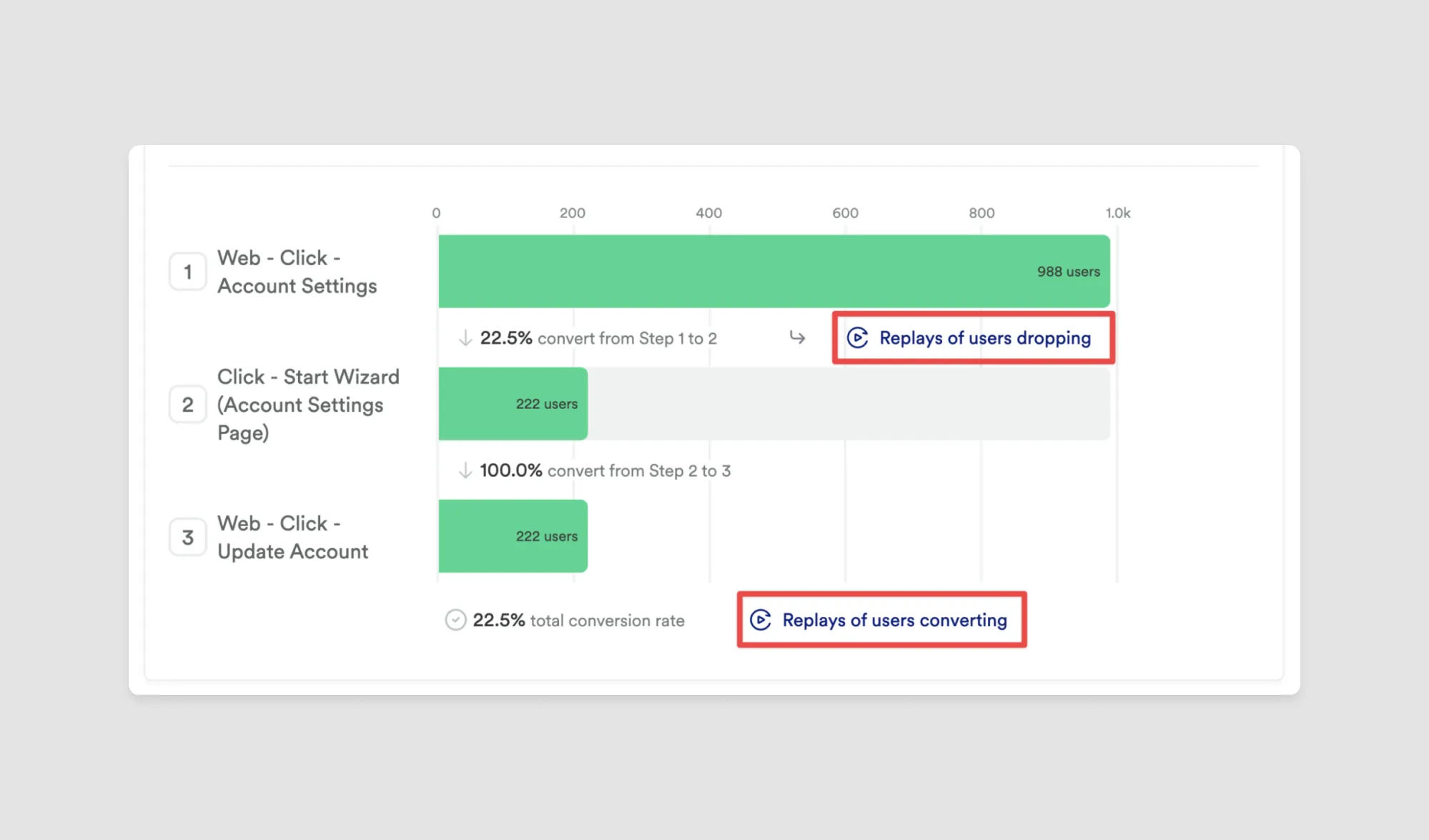
- Predictive analysis: AI models predict user behavior based on historical data, helping prioritize fixes for high-impact areas. For example, Daria Kornienko uses Chat GPT to analyze flow strengths and weaknesses from the user’s point of view. She uploads screenshots of a certain user flow, provides AI with details about the customer and the product, and asks AI to tell what parts of a flow are intuitive or confusing.
Practical tips:
- Use AI-generated insights to guide hypotheses but validate them with qualitative data (e.g., user interviews).
Limitations:
- Analytics data provides the "what," but not the "why." AI cannot determine the motivations behind user actions; conducting quality UX audits requires follow-up research to fill the gaps.
3. Heatmaps and click tracking
Heatmaps and click-tracking tools visualize where users interact with your interface, helping identify areas of confusion or underutilized elements. AI enhances these tools by automating trend detection and providing recommendations.
Use case examples:
- Spotting friction points: Tools like Hotjar and Crazy Egg analyze clicks, scrolls, and interactions to highlight usability problems.

- Generate UX audit reports: Apps mentioned above can aslo process vast amounts of interaction data to generate reports, making it easier to identify areas of interest or neglect.
- Comparing user segments: AI-powered heatmaps like Attention Insight can compare interaction patterns across different user groups, revealing behavior trends.
Practical tips:
- Pair heatmap insights with user testing to verify whether flagged areas genuinely reflect user frustrations.
- Use AI insights to prioritize fixes for high-traffic or critical interaction points.
Limitations:
- Heatmaps show where users click or hover but not why. Behavioral patterns must be interpreted alongside qualitative data to provide meaningful insights.
4. Accessibility audit
Ensuring that your product is accessible to all users is an essential part of any UX audit. AI-powered accessibility tools automatically check compliance with standards like WCAG, flagging issues like low contrast, missing alt text, or improper heading structures.
Use case examples:
- Automated accessibility testing: Tools like Axe DevTools and WAVE scan interfaces for accessibility violations. Some of our designers at Eleken also recommend Figma plugin Attention Insight (mentioned in the heatmaps section) for this purpose. It also shows the accessibility level of the interface.

Practical tips:
- Train your team on accessibility standards to ensure fixes align with best practices.
Limitations:
- Accessibility audits cannot fully account for the lived experiences of users with disabilities. While AI tools detect technical compliance issues, manual testing with real users is necessary to assess practical usability.
Exploring: turning insights into actionable strategies
The exploration stage is where raw research findings transform into actionable strategies. It’s about connecting the dots, generating ideas, and defining solutions that address user needs. Traditionally, this stage relies heavily on brainstorming, task analysis, and competitive research — all of which can be time-consuming and prone to oversight.
AI tools simplify exploration by automating data synthesis, visualizing user flows, and providing strategic insights. They allow researchers and designers to focus on creativity and problem-solving while ensuring the data-driven foundation of their decisions.
Here are some key activities AI can enhance during this stage:
1. Persona building
Creating user personas helps teams empathize with their audience by representing key user behaviors, goals, and pain points. Product design AI tools can analyze user data to develop dynamic, data-driven personas that evolve over time.
Use case examples:
- The most universal way to use AI in creating personas is with ChatGPT. It’s the easiest way because you probably already use it for different purposes. By analyzing provided data such as survey responses, user feedback, or analytics, ChatGPT can synthesize information into detailed and structured user personas. In cases where concrete data is unavailable, plausible hypothetical personas can be generated based on industry trends or target demographics.
For example, below is a person ChatGPT built with the following prompt:
“Build a user persona for a new student engagement app that automates the onboarding and arrival process for university students and agents.”
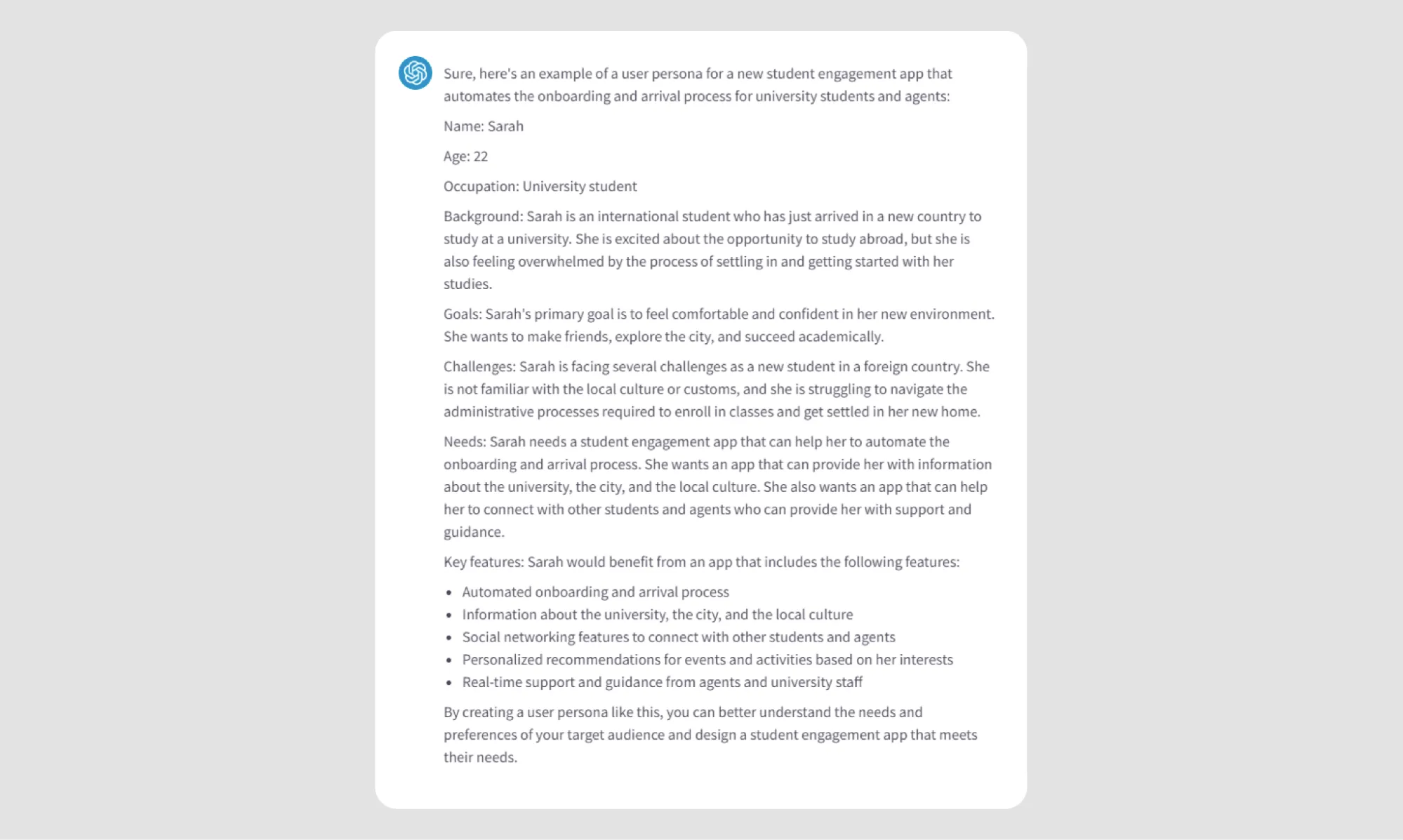
- Persona refinement: AI can identify gaps in existing personas and suggest updates based on new user data. And again, ChatGPT can refine personas by adding depth to motivations, goals, pain points, or behaviors. It can help align personas with specific project needs, such as creating personas tailored for e-commerce, SaaS, or B2B users. Additionally, ChatGPT can assist in anticipating user needs and behaviors by simulating how personas might interact with products or react to specific scenarios.
Practical tips:
- Consider personas as evolving tools — update them as new research or product changes emerge.
- Pair AI-generated personas with storytelling techniques to make them relatable for stakeholders.
Limitations:
- Personas generated solely by AI may lack the emotional depth needed to connect with the team. AI can cluster behaviors into segments, but it doesn’t give you the ‘why’ behind user motivations, which is critical for building empathy.
- Over-reliance on historical data can reinforce biases, leading to inaccurate personas.
2. Task analysis
Task analysis breaks down user workflows into individual steps, helping teams identify bottlenecks and areas for improvement. AI tools can streamline this process by mapping user flows and analyzing usability data.
Use case examples:
- Visualizing user journey map: tools like QoQo.ai can create a structured visualization of a journey map. For example, below is the use case when our designer generated a map based on the user persona right in the Figma file (using information generated by ChatGPT).
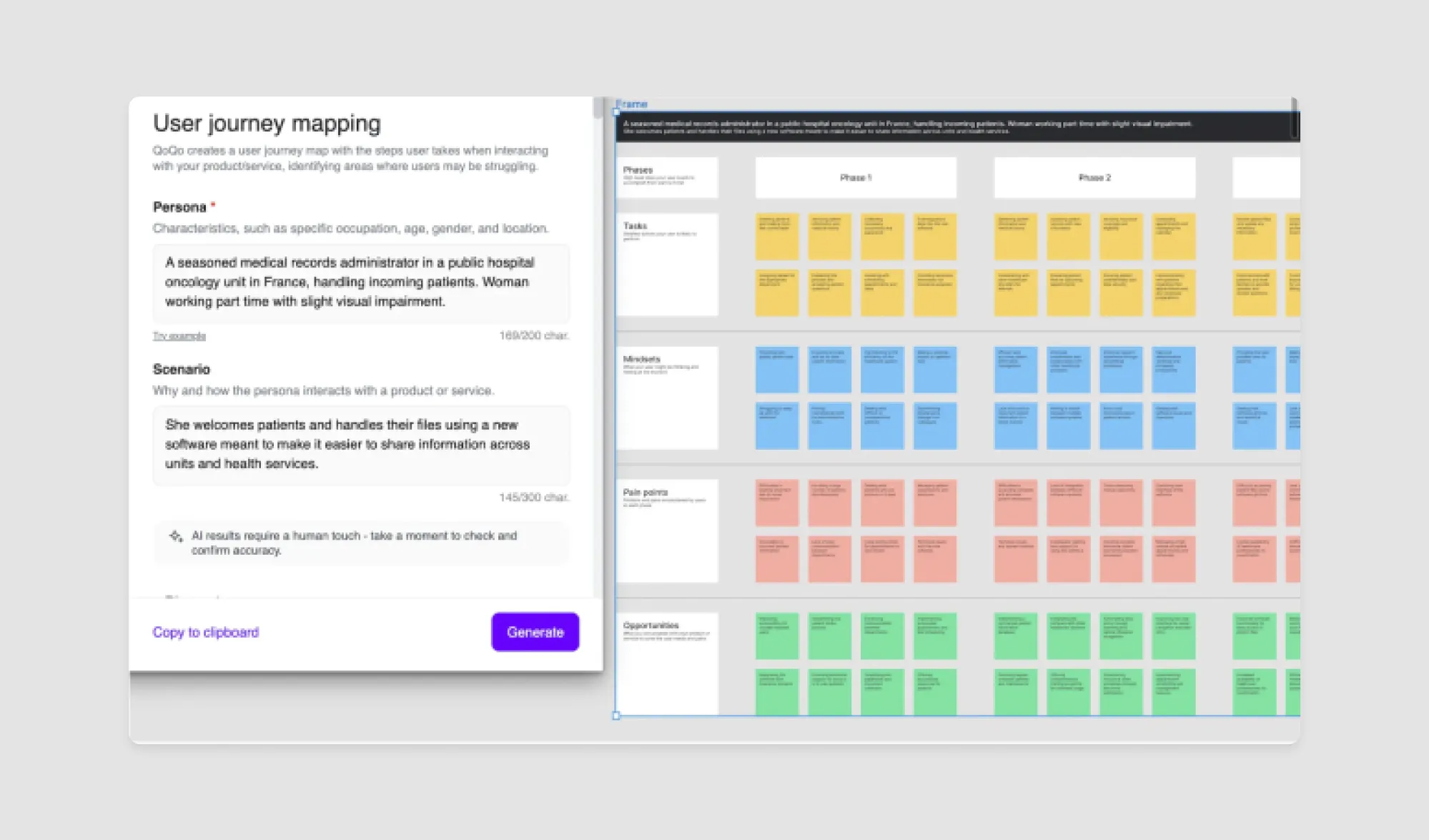
- Visualizing user flows: the same as artificial intelligence can help you with user journey mapping, it can structure features and information into intuitive and logical user flows.

- AI-powered workflow analysis: Eleken designers mention that they sometimes upload screenshots to ChatGPT and ask it to analyze user interactions and define pain points in task flows. However, they also highlight that this method works great at something that gives you food for thought but requires professional human analysis.
- Simulating user behavior: AI tools can predict how users interact with a new design, offering recommendations for improvement.
Practical tips:
- Use AI flow-mapping tools to complement, not replace, manual analysis—this ensures key edge cases are not overlooked.
- Validate AI-identified bottlenecks with qualitative user testing to confirm accuracy.
Limitations:
- AI tools may oversimplify workflows, failing to account for user-specific challenges or edge cases.
- Simulated behaviors may not reflect real-world usage scenarios.
3. Competitive analysis
Implementing AI tools for UX research in this area can dramatically simplify and enhance competitive analysis by automating data gathering, offering actionable insights, and continuously refining strategies with targeted prompts.
While there are many tools available, in practice, we typically rely on one or two that we have access to through a paid subscription. For example, Nazar Neshcheret explains:
For a competitive researcher, I mostly use ChatGPT or Perplexity. I just ask it to find the main competitors and draw up their pros and cons.
The use cases discussed below focus on these tools.
Use case examples:
- Automate data collection and analysis for competitive research. AI can extract competitors' online presence, product offerings, pricing strategies, and generate insights about gaps and opportunities.
Prompt Example:
You are an expert in competitor research. Analyze [competitor website URL] and provide insights into its strengths, weaknesses, and areas of improvement for competitive advantage.
Identify gaps in [competitor website URL] based on usability, content relevance, and engagement features. Suggest three ways to differentiate.
- Create unique selling propositions (USPs) based on gaps and weaknesses. AI can propose differentiators like interactivity, personalization, or value-added services (e.g., webinars, tutorials).
Prompt Example:
"List ways to differentiate our service from [competitor's name] by adding unique features or improving their weaknesses."
- Justify premium pricing through enhanced value. You can ask the tool to emphasize quality, features, and user experience to support higher pricing.
Prompt Example:
"Highlight unique selling points of our product that justify a higher price compared to [competitor]."
Practical tips:
- Create specific and focused prompts (e.g., "Identify the strengths and weaknesses of [competitor website URL]").
- Specify what aspects you want to analyze (e.g., pricing, UI/UX, SEO).
- Break down your analysis into smaller steps, such as first asking for strengths, then weaknesses, and finally for ways to differentiate.
- Start broad and refine based on the outputs. For instance, if the initial result is too general, include qualifiers like "for tech startups" or "specific to mobile responsiveness."
Limitations:
- ChatGPT cannot access real-time data unless paired with a browser or live APIs. This limits its ability to analyze competitors’ most recent activities or updates so you need to pay special attention to the data you can provide to your AI.
- While it can provide general insights, it may not fully grasp niche or highly specific industry contexts without detailed prompts.
- Some outputs might lean towards general best practices rather than being highly tailored to your specific competitive landscape.
4. Prototype testing
Prototyping allows teams to test design ideas early and gather feedback before full implementation. AI tools make this process faster and more iterative by automating feedback collection and analysis.
Use case examples:
- Automatically analyze user interactions with your prototype, identifying usability issues and suggesting improvements without manual effort. Our team often uses Maze for unmoderated user testing, and this tool has great AI features. After testing a new app design, Maze provides a summary highlighting which tasks users found difficult. As well you can use it to test hypotheses.
- Analyze user feedback from open-ended questions to understand how participants feel about your prototype. After asking users for thoughts on the prototype, AI tools like Maze can detect trends like "confusion about navigation" or "positive impressions of the layout."
- Using artificial intelligence to generate follow-up questions for participants based on their interactions or feedback during the prototype test.
- Group users by behaviors, demographics, or responses to tailor insights to specific segments.
- Sketch a layout on paper, upload it to Uizard, and instantly have an editable digital version to refine and test.
Practical tips:
- Clearly define the purpose of your prototype testing. For example, are you testing usability, design aesthetics, or user workflows? Tools like Maze can then be used to create targeted test missions, and Uizard can rapidly generate the required prototypes.
- Use Maze’s heatmaps or Uizard’s attention heatmaps to identify areas where users focus most. This ensures that critical elements, such as call-to-action buttons, are effectively positioned.
- Use the AI features to automate repetitive tasks, like creating wireframes or summarizing feedback, but ensure a human review for nuanced decision-making.
Limitations:
- AI-generated feedback may miss nuanced issues that only emerge during direct user interaction.
- Prototypes tested in isolation may not reflect real-world contexts, leading to misaligned insights.
Testing: validating assumptions with data
The testing stage focuses on validating design decisions by gathering user feedback and measuring product performance. AI tools make this stage faster and more efficient by automating repetitive tasks and analyzing test data with precision. Here's how AI supports key testing tasks:
1. Usability testing
Usability testing uncovers how users interact with a product, identifying areas where they face challenges or confusion. AI enhances usability testing by analyzing user behavior patterns and providing instant feedback.
Use case examples:
- Unmoderated testing: platforms like Maze and Lookback use AI to analyze user interactions during unmoderated tests, providing heatmaps, task success rates, and key behavioral insights.
- Task completion analysis: AI tools track where users abandon tasks, helping pinpoint friction points in workflows.
- Analyzing usability test results and creating a report: Summarize user feedback, identifie recurring themes, and suggest actionable design changes. For example, input user comments into ChatGPT and ask it to highlight common pain points and potential fixes.
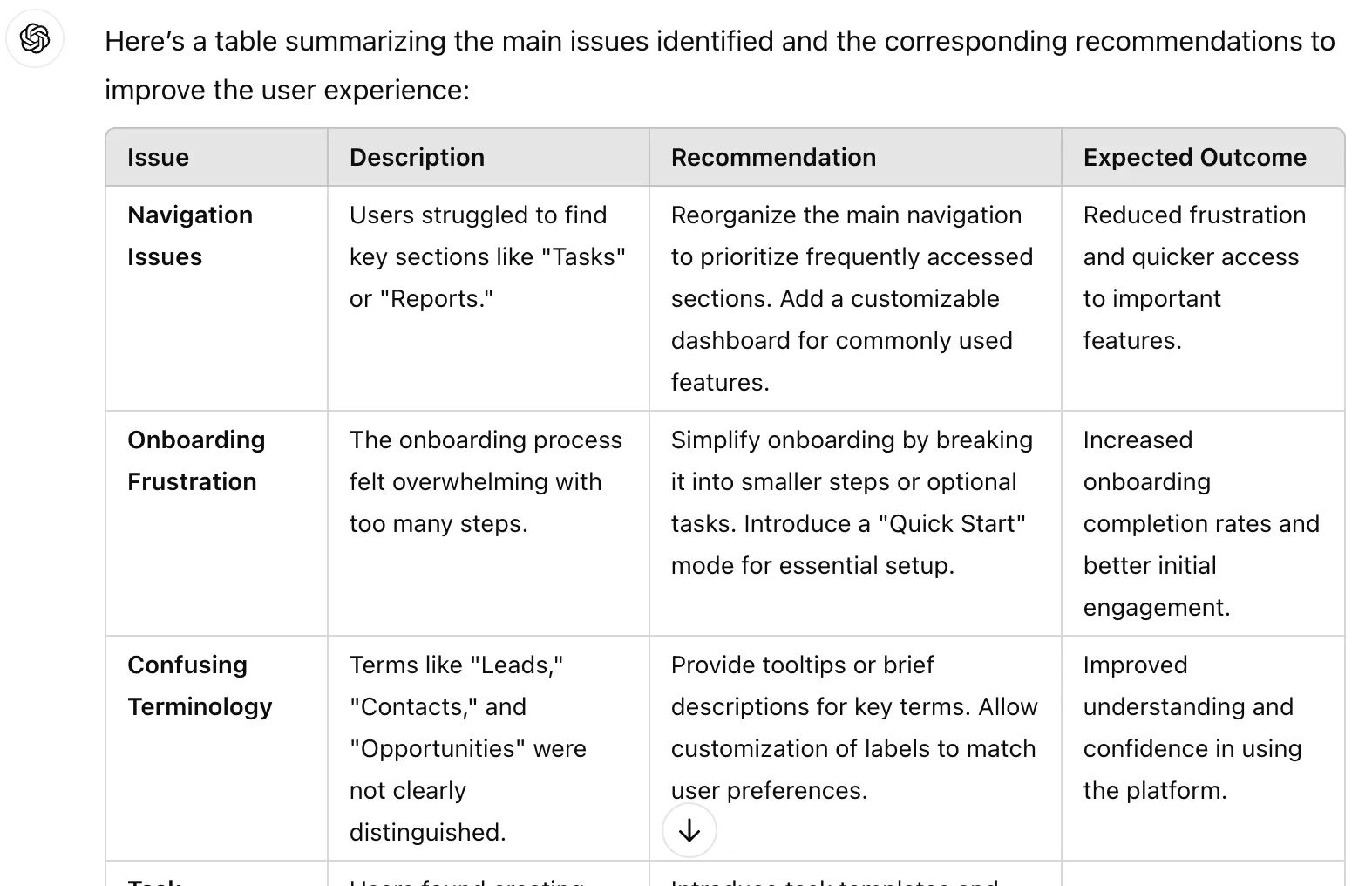
Practical tips:
- Specify what you want to test (e.g., onboarding flow, task completion, error handling) to ensure AI-generated insights are relevant.
- Use AI to evaluate designs against established usability principles like Jakob Nielsen's heuristics. This helps ensure consistency and adherence to best practices.
- Use AI to brainstorm and refine usability testing questions tailored to different stages of the user journey, such as onboarding or troubleshooting.
- Use AI-generated insights as a starting point and complement them with real user testing to validate assumptions and uncover deeper insights.
Limitations:
- AI cannot physically interact with your product (e.g., touchscreens, hardware) or experience real-world environmental factors like connectivity issues.
- Using AI feedback for research, you may miss edge cases or specific use cases unique to your target audience or industry.
2. Benchmark testing
Benchmark testing evaluates your product’s usability against competitors or industry standards. AI helps automate benchmarking by comparing performance metrics such as task completion times, user satisfaction scores, and error rates.
Use case examples:
- Performance comparisons: Platforms like UsabilityHub provide AI-generated benchmarking reports, showing how your product stacks up against competitors.
Customer satisfaction analysis: AI tools can analyze NPS (Net Promoter Score) feedback, identifying common themes in positive and negative responses.
Practical tips:
- Set clear benchmarks for success before testing begins, such as task completion rates or satisfaction scores.
- Use AI findings to identify competitive gaps but validate them with qualitative feedback.
- Benchmark regularly to track progress and adapt to changing industry standards.
Limitations:
- Benchmarks provide relative comparisons but may not capture unique aspects of your product that matter most to users.
- AI-generated insights can miss context-specific challenges that qualitative research could uncover.
Listening: gathering feedback to drive iteration
The Listening stage focuses on collecting and analyzing user feedback to refine the product and address ongoing issues. Usually, such a process requires processing large volumes of data, and that’s exactly where artificial intelligence can be very helpful.
1. Surveys
Surveys are one of the most straightforward ways to gather user feedback. AI in UX research can create questions, analyze responses, and detect patterns that may otherwise be missed.
Use case examples:
- Input your research goal to generate relevant survey questions tailored to your objectives. To understand user satisfaction, you might input "measure user satisfaction," and the AI will produce appropriate questions to gather insights.

An example of Hotjar creating an AI-generated survey
- The AI evaluates open-ended responses to determine user sentiment, categorizing feedback as positive, negative, or neutral.
- AI detects recurring themes in responses, applying tags to categorize feedback efficiently. Tags like "navigation issue" or "feature request" help identify common user concerns.
- AI, for example, Hotjar, compiles survey responses into structured reports, including key findings, supporting quotes, and actionable recommendations.
Practical tips:
- Pair AI-analyzed qualitative feedback (e.g., sentiment analysis) with quantitative survey data (e.g., satisfaction scores) for a more comprehensive understanding.
- Use AI tools to prioritize essential questions, reducing survey length to maintain user engagement.
Limitations:
- AI-generated questions may lack the specificity required for unique research objectives, requiring human refinement.
- The quality of AI outputs depends heavily on the clarity and detail of the prompts or initial survey goals provided.
2. Search-log analysis
Search logs are an often-overlooked source of feedback, offering valuable insights into user intent and pain points. AI tools help analyze search queries to identify patterns, common themes, and gaps in content or functionality.
Use case examples:
- Detect frequent searches like "product installation guide" that return no results. This indicates a need to create or improve related content.
- Identify users frequently misspelling terms or searching with synonyms (e.g., "sofa" vs. "couch") and recommends enhancing the search engine with auto-correct or synonym handling.
- Cluster search terms to reveal emerging interests, such as increasing searches for "eco-friendly products."
Practical tips:
- Regularly review search logs to stay on top of changing user needs.
- Pair search log insights with survey data or direct user feedback to validate findings and prioritize fixes.
- Use clustered insights to adapt your offerings or marketing campaigns to align with user trends.
- Pair search-log insights with survey or usability testing data to confirm user needs and frustrations.
Limitations:
- Search logs reveal what users are looking for but not why they are searching for it. Follow-up research is required to uncover the motivations behind search behavior.
3. Analytics review
Analytics tools provide valuable insights into how users interact with your product, such as where they spend the most time, where they drop off, and what features they engage with. As most of us have access to ChatGPT, let’s talk about how this tool may simplify your work here.
It can analyze, interpret, and summarize analytics data to extract valuable insights during UX research. While it cannot directly access analytics platforms, you can input data or summaries for it to process. Here's how ChatGPT can help:
Use case examples:
- Analyze metrics like session duration, bounce rates, and click-through rates to identify how users interact with your site or app.
Example prompt: "Here's user engagement data: [Insert metrics]. Identify trends and suggest ways to improve user retention."
- Spot pages with the highest or lowest traffic, conversions, or engagement.
Example prompt: "Given this traffic and conversion data: [Insert data], which pages should we prioritize for optimization?"
- Analyze funnel data to find where users abandon processes (e.g., sign-ups or checkouts).
Example Prompt: "Here is our checkout funnel data: [Insert data]. What do you see as the major drop-off points, and what can we do to address them?"
- Provide insights into how different user segments (e.g., by device, geography, or behavior) perform.
Example Prompt: "Analyze this data segmented by device type: [Insert metrics]. What differences exist, and how can we optimize for mobile users?"
- Condense large analytics reports into key takeaways for stakeholders.
Example Prompt: "Summarize this analytics report: [Insert data]. Highlight trends, anomalies, and next steps."
Practical tips:
- Clearly describe the data you're inputting (e.g., user behavior, traffic sources, or funnel performance) to help ChatGPT generate accurate insights.
- Instead of feeding all analytics data, focus on key metrics (e.g., bounce rates, conversion rates, session duration) for targeted feedback.
- Use visualization tools (e.g., Google Analytics, Tableau) to prepare data summaries, then provide those summaries to ChatGPT for interpretation.
Limitations:
- ChatGPT cannot directly access analytics platforms like Google Analytics or Hotjar. You need to manually input data for analysis.
- ChatGPT can only analyze static or pre-processed data, so it’s not suited for real-time monitoring or dynamic dashboards.
- Without detailed context, ChatGPT may misinterpret metrics or offer overly generic recommendations.
From insights to action: how AI transforms UX research
Definitely, AI has become a game-changer in UX research, streamlining workflows, automating repetitive tasks, and uncovering actionable insights faster than ever. For many of us it works like a personal junior assistant. Yet, we can’t help but mention once again that AI is a tool — not a replacement for human intuition and expertise. At each stage of the UX research process, we’ve discussed the benefits of AI’s capabilities, but the best results come from combining these technologies with thoughtful, human-driven research.
To sum up all the information, let’s quickly recap how AI can be applied to the key stages of UX research:
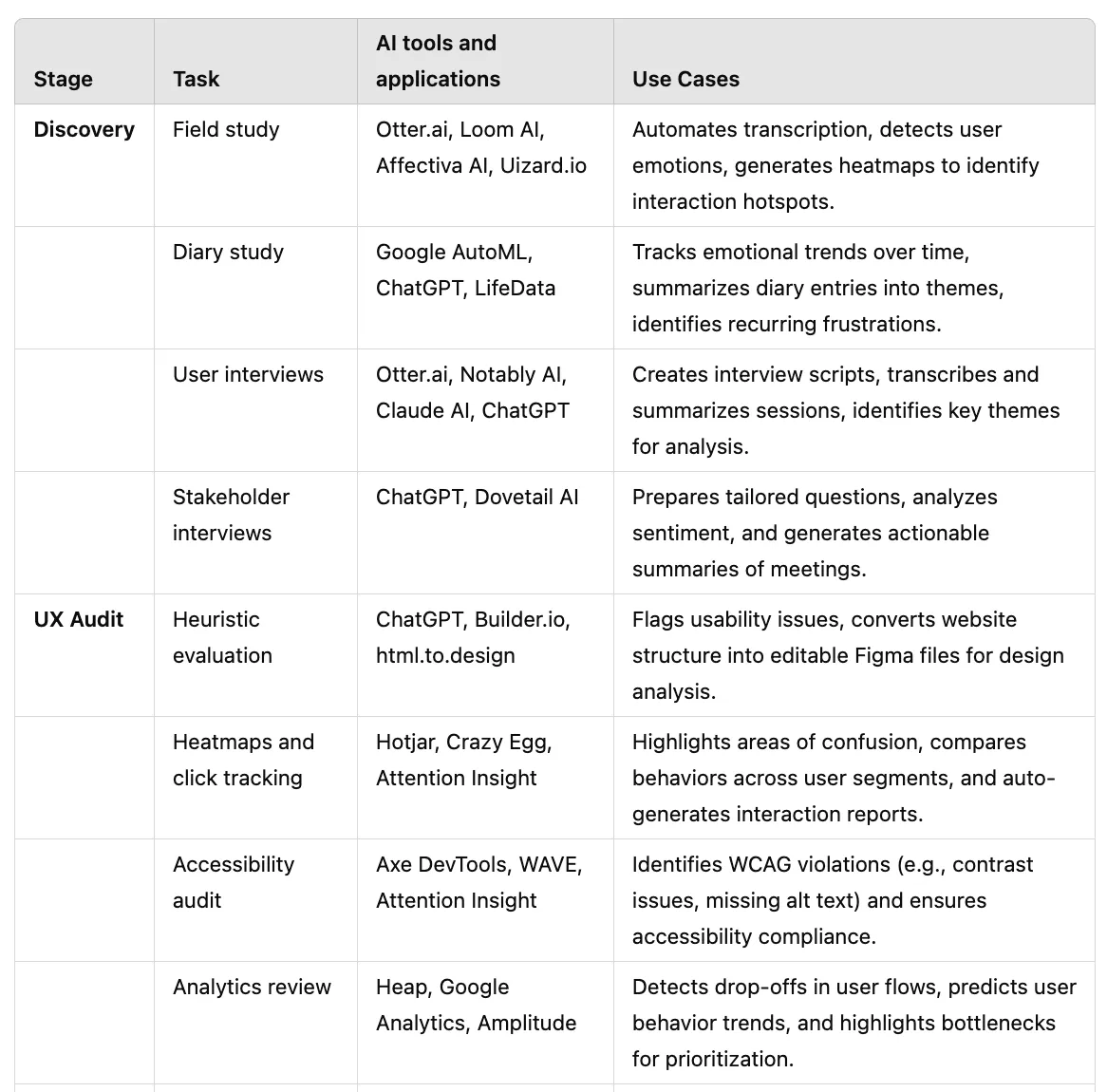
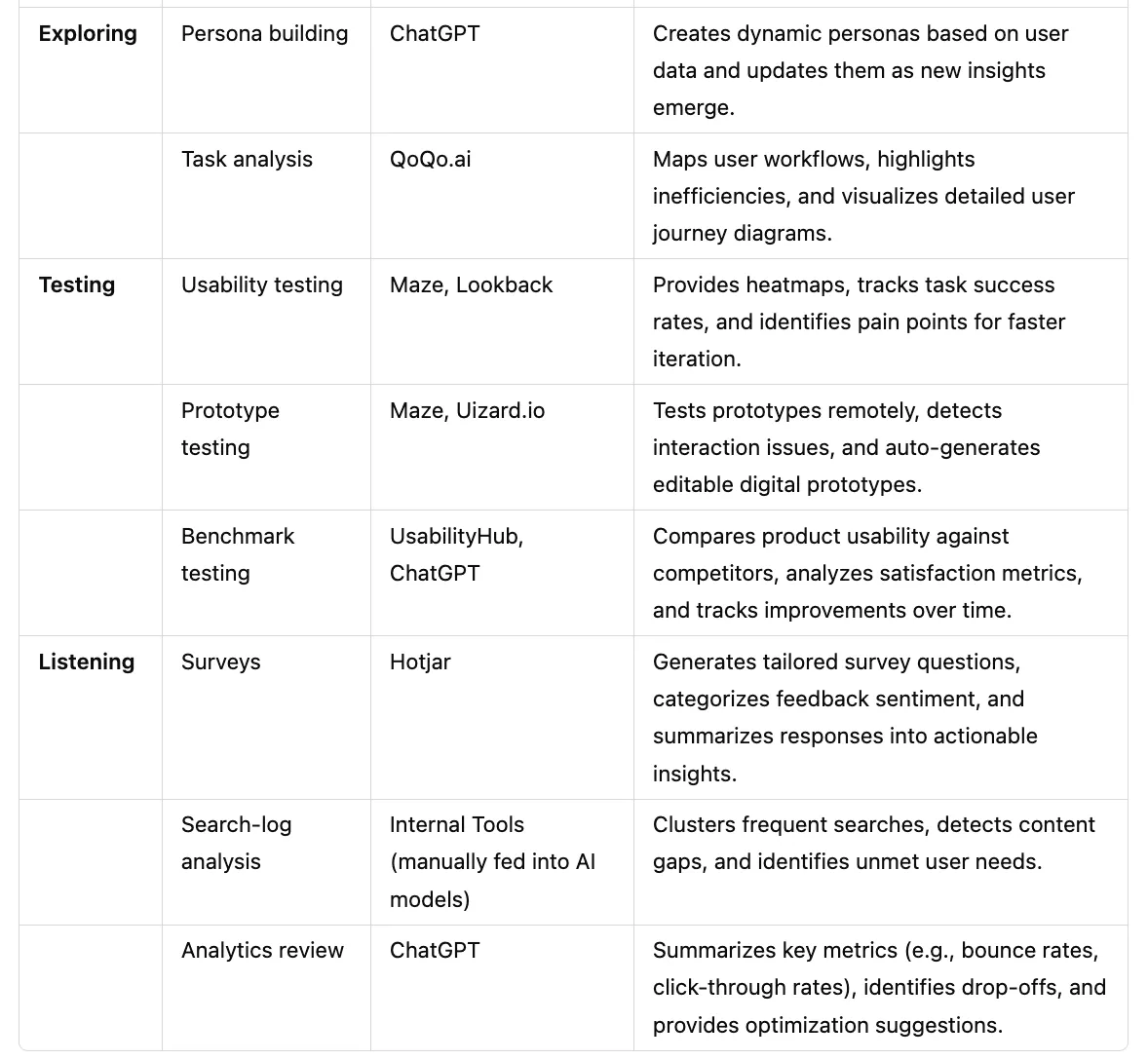
The last thing we want to say here is – it’s definitely worth integrating AI into your product, your work processes, and your business. Good luck with conquering this exciting new frontier!